Graduate School of Science and Engineering Information and Computer Science
- Course Outline
- Laboratory for Information Theory and its Applications
- Information Systems Laboratory
- Intelligent Information Processing Laboratory
- Intelligent Mechanism Laboratory
- Intelligent Systems Design Laboratory
- Socio-informatics Laboratory
- Co-Creation Informatics Laboratory
- Applied Media Information Laboratory
- Network Information Systems Laboratory
- Intelligent Mechatro-Informatics Laboratory
- Spoken Language Processing Laboratory
Laboratory for Information Theory and its Applications
Website of the Laboratory 【In Japanese】We invite you to join us to do research and development on high-speed, reliable, robust and secure communications
Staff

JUN CHENG
[Professor]
Acceptable course | |
---|---|
Master's degree course | ✓ |
Doctoral degree course | ✓ |
Telephone : +81-774-65-6295
jcheng@mail.doshisha.ac.jp
Office : YE-215
Database of Researchers

KIMURA Tomotaka
[Associate Professor]
Acceptable course | |
---|---|
Master's degree course | ✓ |
Doctoral degree course |
Telephone : +81-774-65-6294
tomkimur@mail.doshisha.ac.jp
Office : YE-214
Database of Researchers
Research Topics
- Wireless communications for IoT
- Machine Learning for Wireless Communications
- Coding for multiple-access channel
- Coding for MIMO systems
- Adaptive signal processing for wireless communications
- Channel coding and wireless communications
- Robust wireless netwrorking
- Communication network analysis
- Network architecture design
Research Contents
Information theory, coding theory, communication theory, teletraffic theory and cryptography are fundamentals in constructing modern information network. Based on these basic theories, we do research and development on information transmission, with particular attention to reliable communications with channel coding and robust communications with teletraffic theory. We expect this focus to bring new and important scientific and applied knowledge to information transmission.
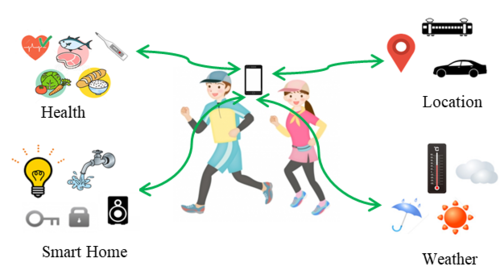
Wireless Communications for IoT
The Internet of Things (IoT) bring wireless connectivity to anything, from tiny static sensors, machines to
robots, vehicles and drones. A successful implementation of IoT calls for wireless technologies that are able to
support a much larger number of connected devices, and that are able to fulfill much more stringent requirements
on latency and reliability than current systems. A generic scenario in IoT involves a very large number of idle
(inactive) devices, but in a typical application, only a small (unknown) subset of devices are active at any given
instant. In this scenario, short packet are the typical form of the traffic with the need of low latency. The
classical information-theoretic results of a K-user multiple-access, however, are not applicable, since the
assumptions of sustained connectivity and sufficiently large lengths of packets are not valid.
This research will develop and put forward a random-access strategy with short-packet transmission to meet the new
requirements of IoT networks. Random access can support a very large number of devices to efficiently communicate
in a sporadic and uncoordinated way. Some recent advances from information theory, e.g., the finite-length random
coding bounds for the multiple access channel, provides us new information-theoretic metrics for short-packet
transmission. Such leverage tools from coding theory as code on sparse graph, extrinsic information transfer
charts, and message passing decoding will be utilized for the design of the novel multiple access paradigm and its
analyses.
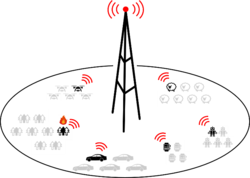
An example of IoT network with a very larger number of devices, e.g., sensors for fire detection, vehicles, drones, robots etc..
Machine Learning for Wireless Communications
Recently, machine learning demonstrates a great advantage in solving optimization problems. A prominent advantage
is its pure data-driven method, where the networks/systems are optimized over a large training data set, while the
conventional model-driven method attempts to capture knowledge and derive decisions through explicit
representation and rules in mathematics.
This project will focus on the application of machine learning to wireless communication systems, especially to
signal detection and channel decoding over a class of uncertain/variable wireless channels. Machine learning in
wireless communication systems has an advantage that it is easy, in computer simulations, to collect the large
training dada set with automatic classification labelling of target data. Since the conventional probability
models of the wireless channels have a gap between these models and real-world physics, the data-driven machine
learning is potential way to close the gap, and thus enhance the robustness of wireless communications systems.
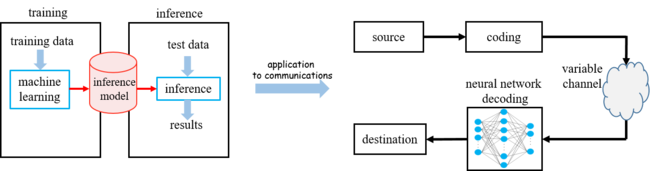
An example of application of machine learning to wireless communications systems
Channel coding and decoding algorithms for wireless communication systems
In wireless communication systems, channel noise and interference would result in the unreliable information
transmission. The interference, for example, in multiuser communication systems, is due to that multiple users
share a common channel. In multiple-input multiple-output (MIMO) communication systems, the interference occurs
due to simultaneous transmission from multiple antennas. Shannon’s coding theorem shows that the reliable
information transmission is possible if the transmission rate is less than the channel capacity. We focus on the
channel coding to realize the reliable information transmission in multiuser communication systems and MIMO
systems. We aims high-reliable coding schemes and low-complexity decoding algorithms with the transmission rate
approaching the theoretic limit. We will publish the results in leading international journals and conferences,
and will transfer results from recent research in these areas to industry in mobile communications, wireless LAN,
IoT, and satellite communications.
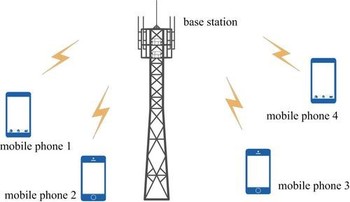
An example of multiple-access communications in mobile networks
Robust wireless networking
In general, wireless communications are inferior to wired communications (e.g., optical fiber communications) in
terms of stability. We thus study robust and reliable networking technologies to overcome various failures that
occur in wireless networks. Especially, we consider sparse mobile ad-hoc networks, where the node density is very
sparse. In conventional wireless networks, relay nodes use Store-and-Forward transmission to forward information.
Specifically, when a relay node receives a packet from an adjacent node, the relay node forwards the packet as
soon as possible. However, in sparse mobile ad-hoc networks, this method cannot be adopted because there are no
neighbor nodes for most of the time. Therefore, Store-Carry-Forward (SCF) routing has been proposed for delivering
messages. In SCF routing, when a node receives or generates messages, it stores them in its buffer. The node then
carries the messages until it encounters other nodes. When this happens, it forwards the messages to the
encountered nodes. By repeating this procedure, the messages are finally delivered to the destination node. So
far, we have analyzed the performance of the existing SCF routing schemes, proposed some efficient SCF routing
schemes, and realized various network services using SCF routing schemes.
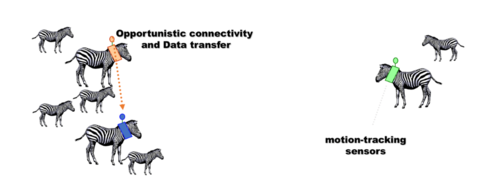
An example of sparse mobile ad-hoc networks
Keywords
- Wireless communications
- Internet of Things
- Machine Learning
- Error-correcting code
- Signal processing
- Communication network analysis
- Network architecture design
- Robust Wireless Networking